Cutting up shoes to measure their footprint
Table of contents
Our mission at Carbonfact is to measure the environmental impact of clothes. This involves a lot of steps. The main one is to determine what materials a product is made of, along with each material’s mass. This is straightforward for most clothes like jumpers and pants. These are typically made of a single fabric, such as cotton or polyester. The mass of each material is roughly the same as the product’s mass.
Footwear products are a different beast, mainly because they contain many parts. For instance, the sneakers we measure contain anywhere between 30 and 80 individual parts. Some parts are found in most shoes (e.g. outsoles, vamps, laces), while others are specific to some shoes (e.g. shanks, gussets, mudguards).
Each part in a shoe is made of a different material, such as rubber, leather, or plastic. A shoe’s mass is not evenly distributed across its parts. For instance, outsoles are often the heaviest part. They are commonly made of rubber, which usually has the largest environmental impact.
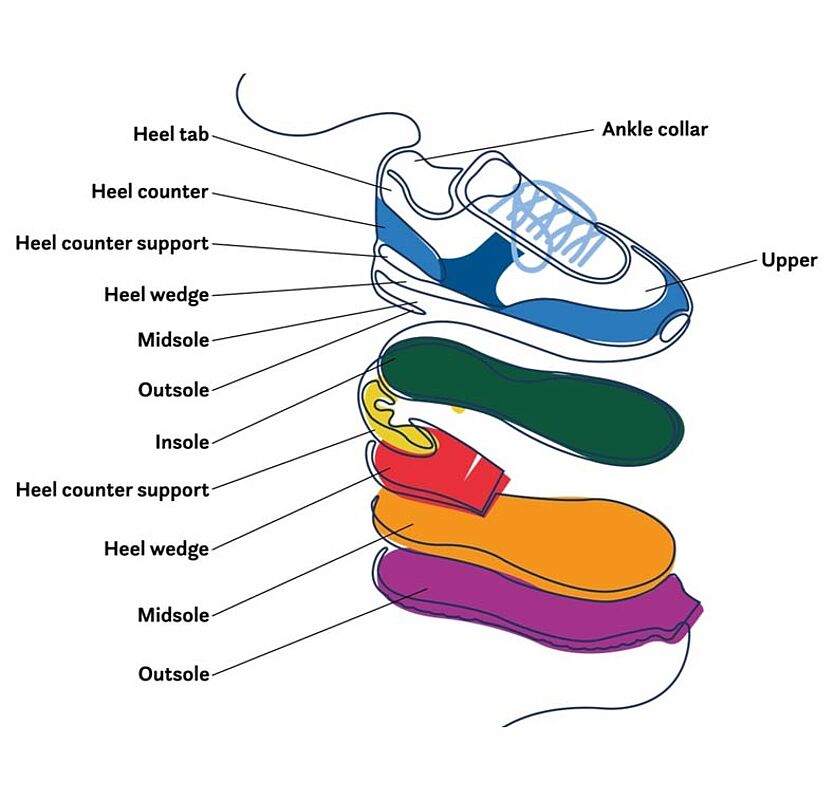
Measuring the environmental footprint of a pair of shoes is difficult for a couple of reasons.
First, there is no agreed-upon taxonomy of shoe part names. Each customer we work with uses a different one. And in most cases they’re not even using a taxonomy: it’s just a bunch of free text fields. Here’s a word cloud that gives an idea of the variety of names we encounter.
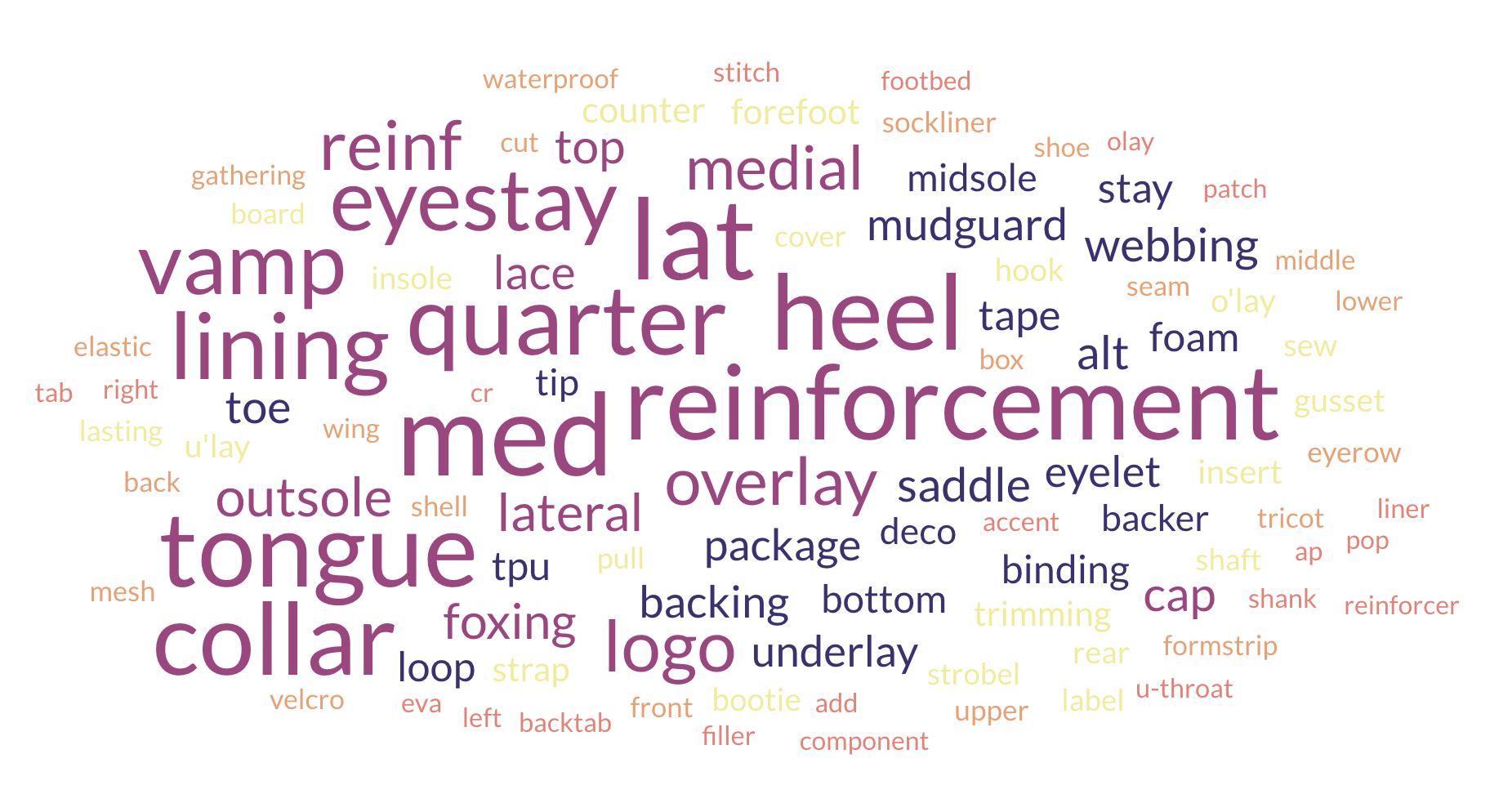
Second, footwear companies don’t always know the mass of each part. They might know the mass of the product, because that is required for shipping and inventory management. This is a problem because a shoe can contain several materials, spread across different parts. The mass of each material has a direct impact on the product’s environmental footprint.
This second problem can be alleviated by addressing the first one. We could build a database of shoe parts with their corresponding mass, when the latter is available. In case the mass of a part in a new pair of shoes isn’t available, we could look up the typical mass of the given part in the database. This requires mapping the free text part names to an agreed-upon taxonomy.
Mapping free text names to a taxonomy is a classification task. We could use a machine learning model to do this. Alas, this requires having a taxonomy available, which we don’t have. The modern way to circumvent this could be to use clustering to bucket the free text names into groups. We could then use zero-shot classification to build a training set for a classification model. Too complicated.
What about doing it the old-fashioned way? That’s what we did during our latest work retreat at Carbonfact. We got together in a room and did a card sorting exercise. The part names were printed out on pieces of paper that we layed out on a long kitchen table. Everyone took part and sorted the papers into groups, which we defined together on the fly. We recorded the taxonomy and the mapping in a shared spreadsheet.
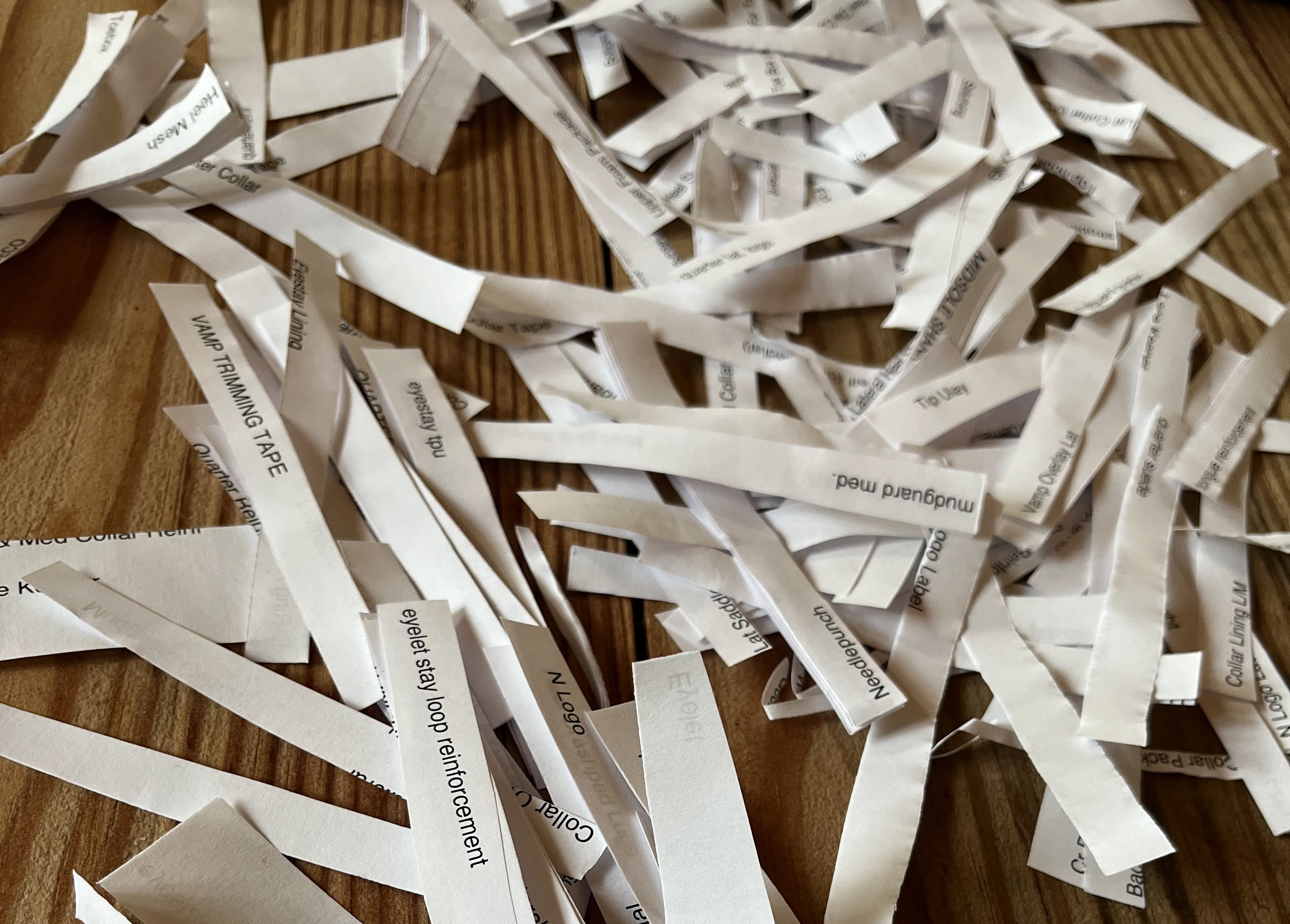
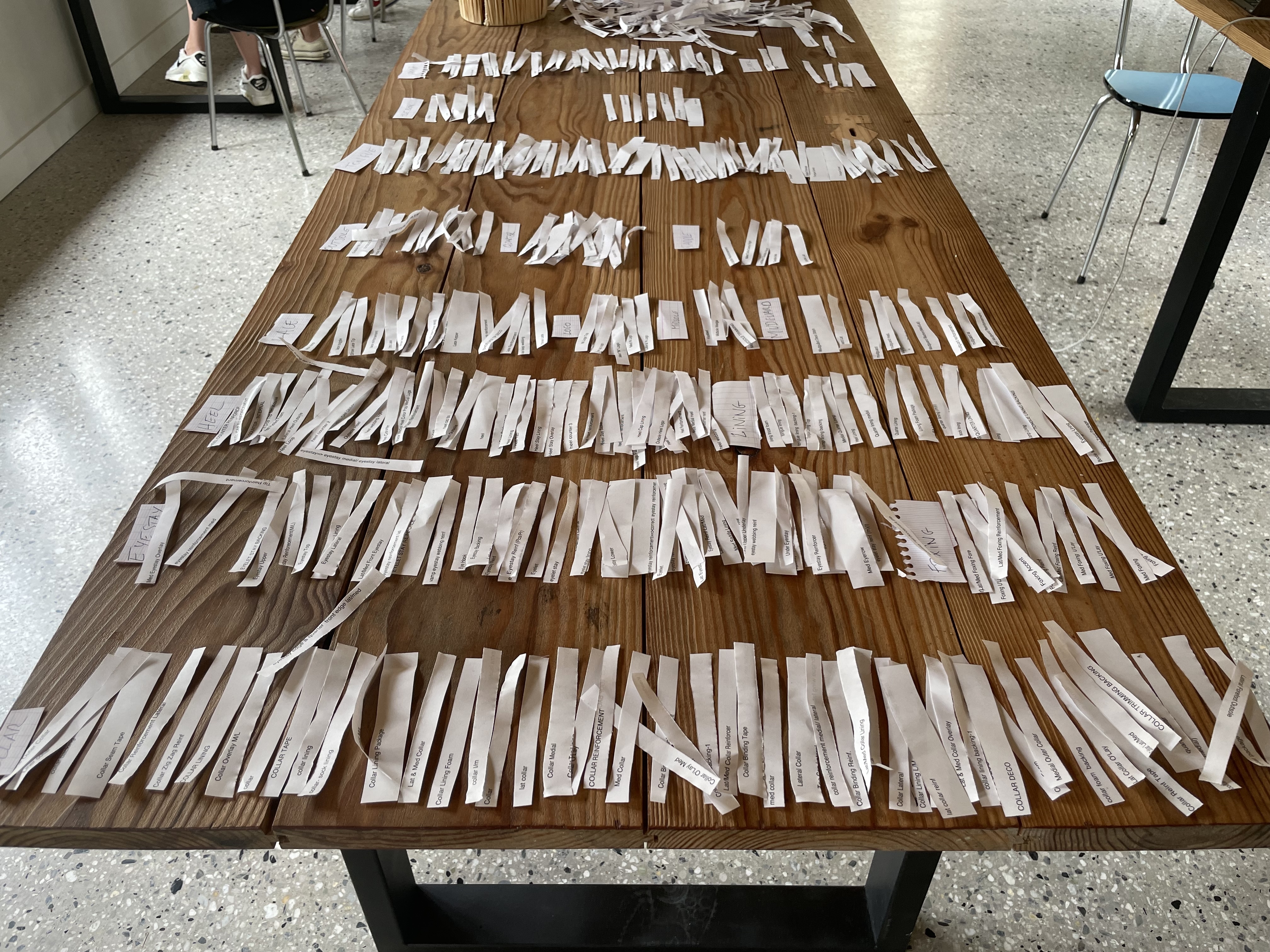
After a few iterations, we reached a list of roughly fifty part names, which were organized in a hierarchical taxonomy:
insole -> BOTTOM/INSOLE
insert -> BOTTOM/INSOLE/INSERT
insole lining -> BOTTOM/INSOLE/LINING
outsole -> BOTTOM/OUTSOLE/BODY
heel -> BOTTOM/OUTSOLE/HEEL
...
welt -> FOXING
lace -> LACES
aglet -> LACES/AGLET
A hierarchical taxonomy is useful because it allows handling parts with different levels of granularity. For instance, sometimes the mass is available for the bottom part of the shoe as a whole, but not for the outsole and the midsole separately. To me this is a good example of Postel’s law: be conservative in what you do, be liberal in what you accept from others.
The next step was a bit more fun. We grabbed some cutters and scissors and took shoes apart. A fair amount of us brought an old pair of shoes with them to the retreat, which gave us a variety of sneakers and boots to work with. We cut up each shoe and weighed every part using a kitchen scale. We then assigned each part to a group in the taxonomy. This resulted in a valuable dataset of shoe parts with their masses.
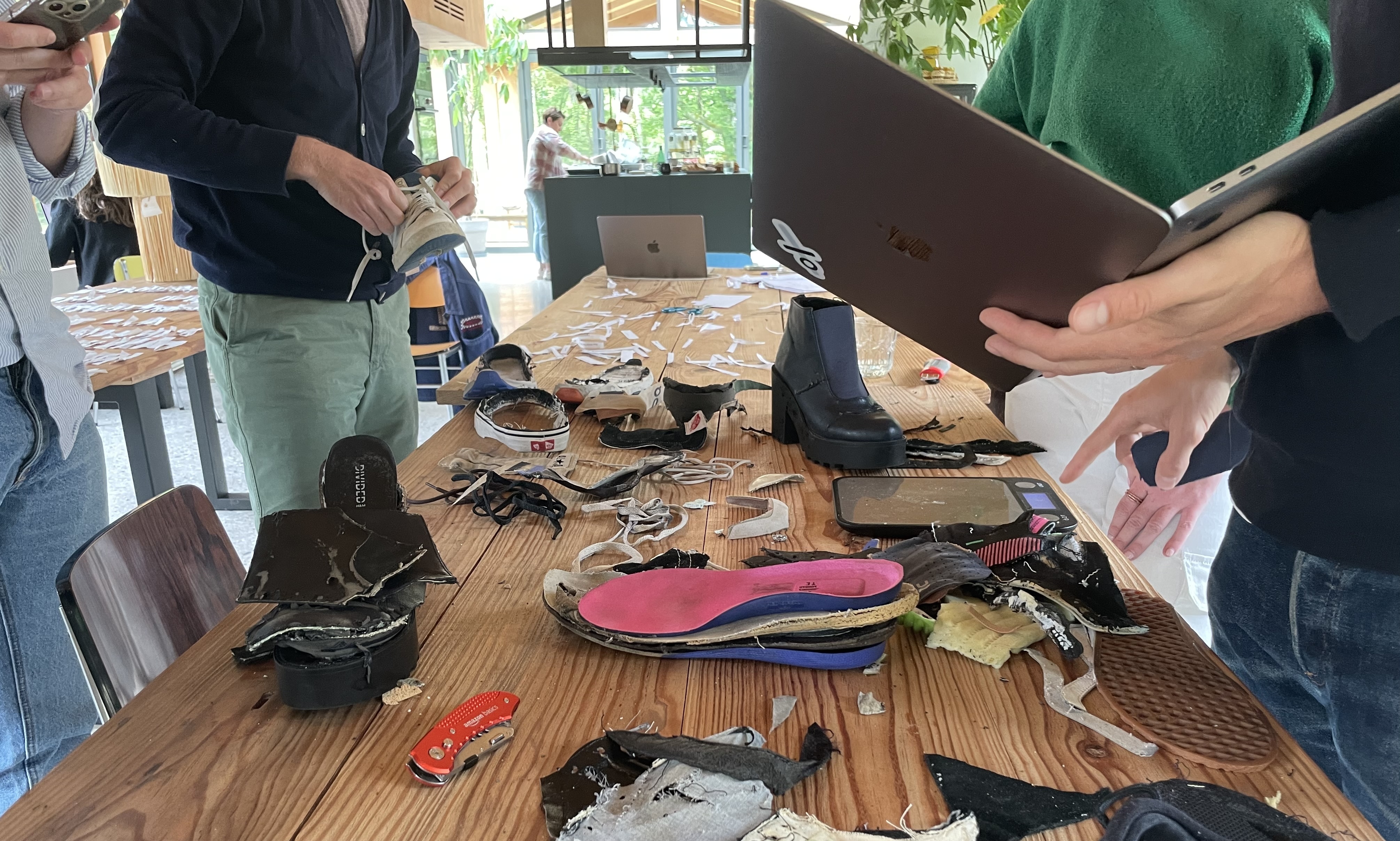
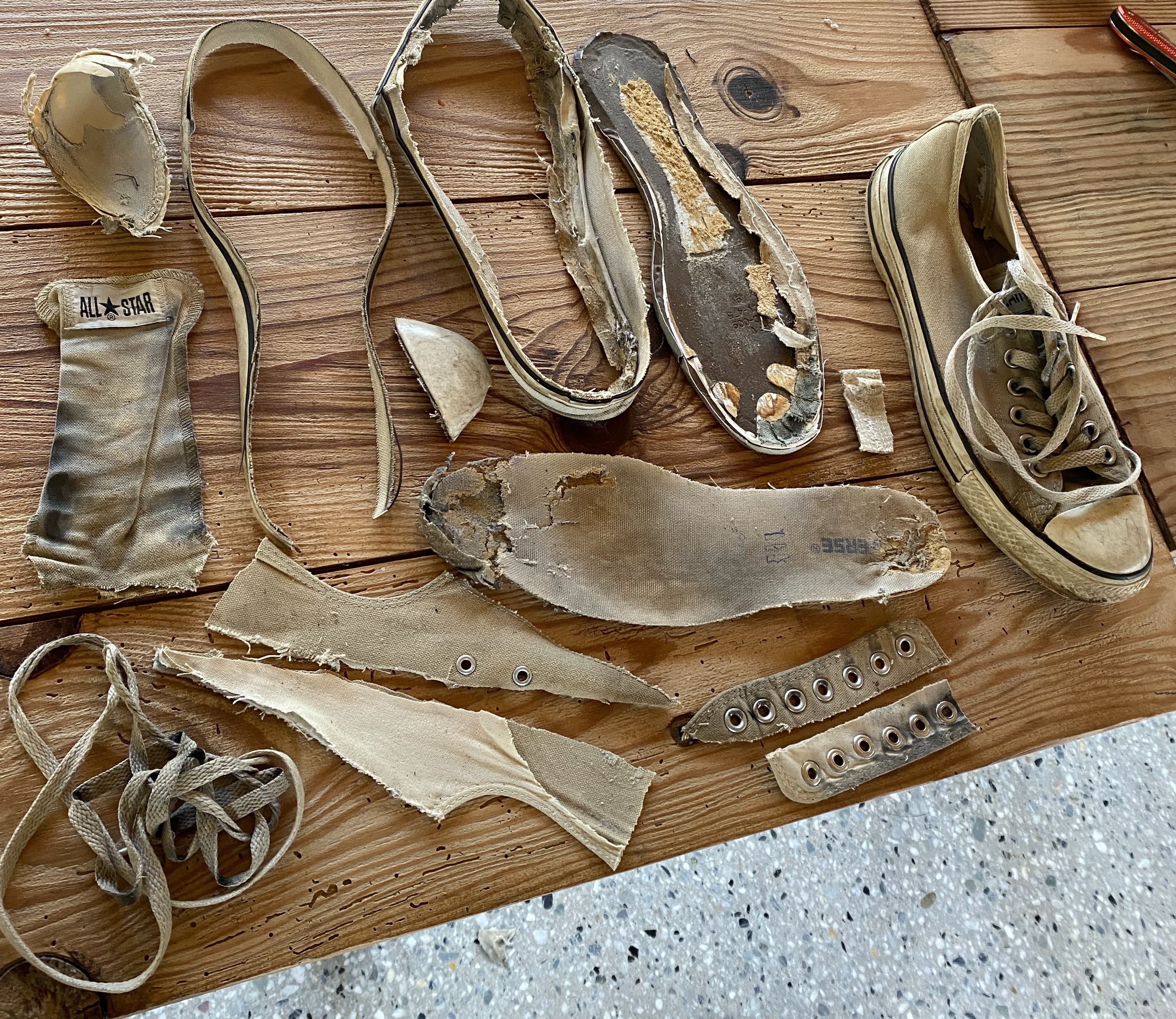
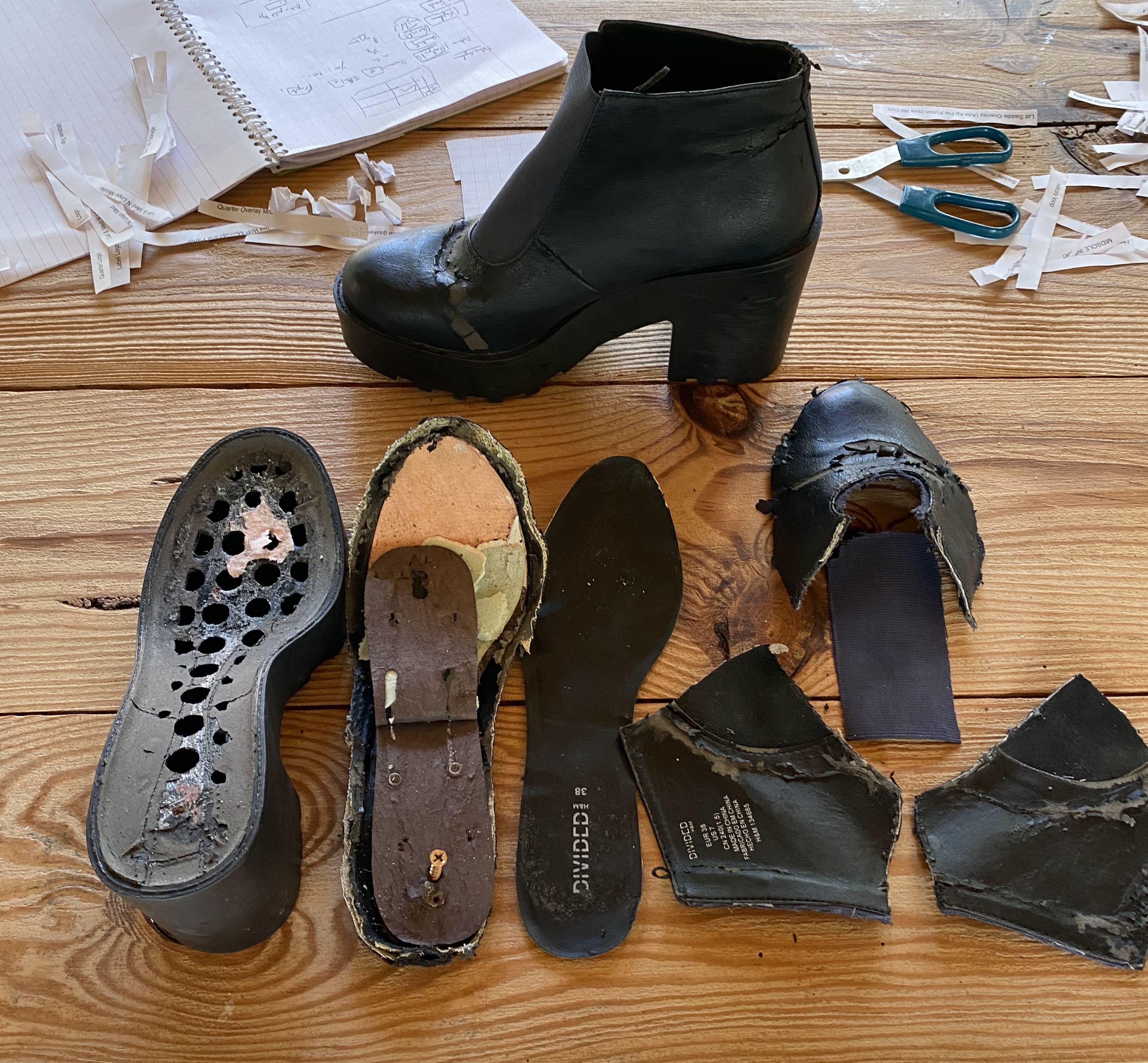
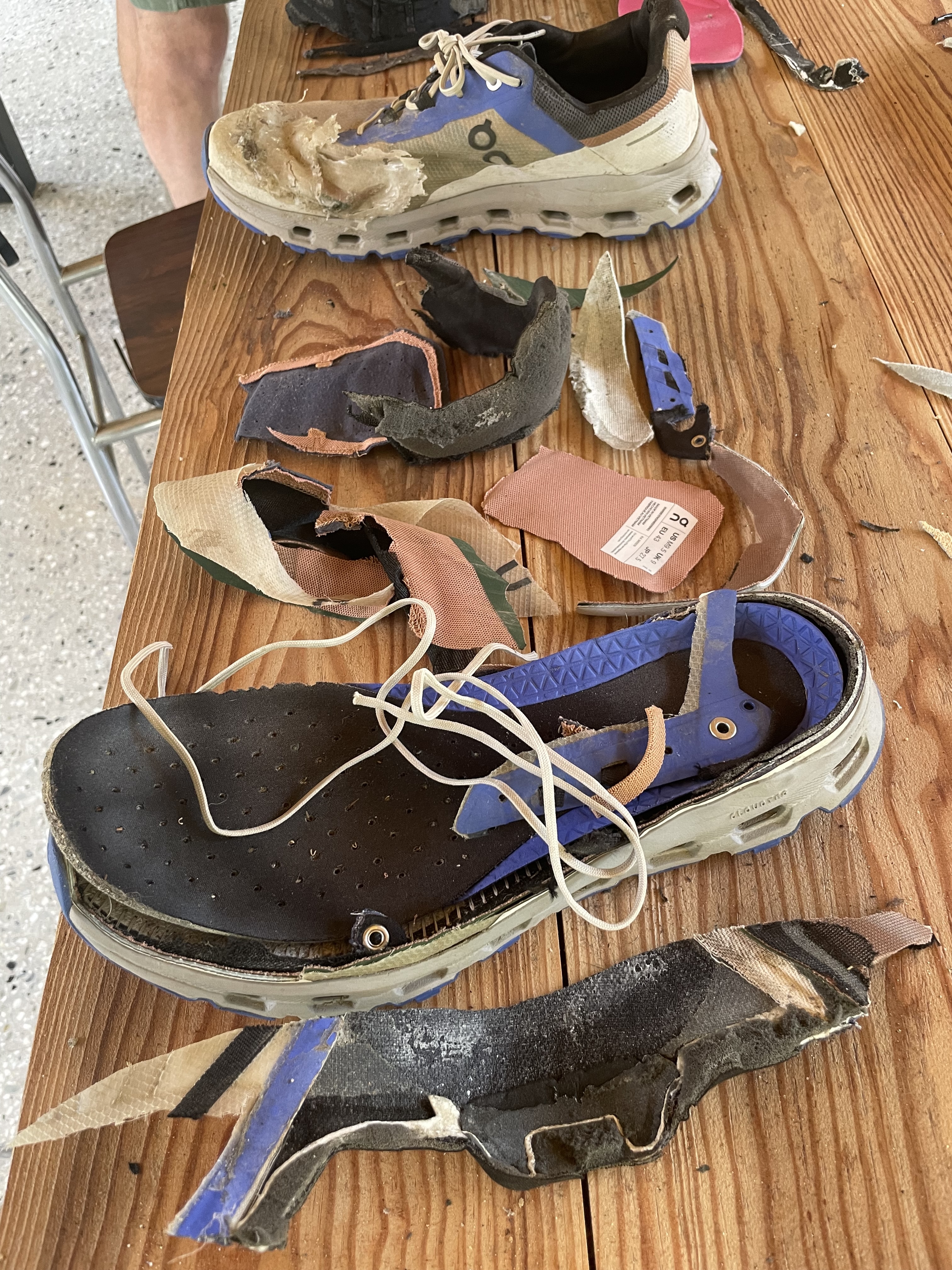
This workshop was a good investment of our time. In practice, it’s going to make our footwear data model much more robust. We can now map free text part names to a standardized taxonomy, which in turn allows us to handle each part in a bespoke manner. I will be able to leverage the work we did to build a machine learning classifier in order to scale the process. Our footwear customers will massively benefit from this.
As a data scientist, I spend most days manipulating data with my computer. It was refreshing to do something physical, by actually touching and manipulating the actual objects behind the data. It was also a great team-building exercise. We had a lot of fun cutting up shoes and discussing the best way to group them. I can’t wait for the next retreat!